Plenary session explores the potent, dynamic terrain of artificial intelligence applications in oncology
//
Estimated Read Time:
Advances in artificial intelligence (AI) have captured the public imagination and fostered transformative changes across sectors, from art to medicine and disease diagnosis to drug discovery.
The plenary session AI at the Interface: Accelerating Evidence Generation, Advancing Disparities Research, and Improving Trial Design on Wednesday, April 10, at the American Association for Cancer Research Annual Meeting 2024 delved deeper into the evolving landscape of AI tools in oncology.
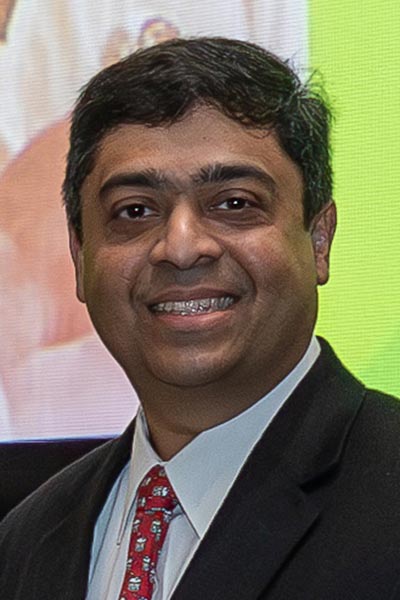
The session is available on demand for registered Annual Meeting attendees through July 10, 2024, on the virtual meeting platform.
The session chair, Vivek Subbiah, MD, chief of early-phase drug development at Sarah Cannon Research Institute, said, “The future is now.” He said that for a broad audience, AI represents a world of sophisticated computer programs that “learn” from data to make decisions.
“Here, today, April 2024, we are with AI at the interface,” he said. “We envision a future for democratizing clinical research and clinical trials.”
Potential and considerations around AI use in medical imaging
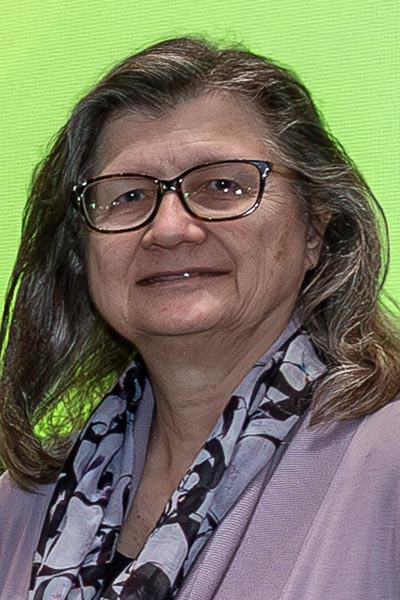
Maryellen L. Giger, PhD, A.N. Pritzker Distinguished Service Professor of Radiology at the University of Chicago, highlighted important considerations when implementing AI-based approaches for clinical imaging: diverse datasets needed to train and generate trustworthy AI systems; understanding how end users will employ AI; AI system and user responsibilities; and how “off-label” AI use differs from off-label therapy.
Giger said that AI-assisted radiologic breast cancer screening “has shown very impressive progress.” She discussed how AI systems, such as computer-aided diagnosis (CADx), can now perform “virtual biopsies” based on radiologic data and aid in prognostication of cancer likelihood and aggressiveness.
Giger described AI applications in cancer imaging in helping predict outcomes or treatment response and in combining imaging data with transcriptomics data to identify associations between gene expression and tumor features.
Giger said, “Good data is the new oil—data is important. [Data] needs to be curated, diverse, representative, and reusable.” She reviewed the inception of and resources available at the Medical Imaging and Data Resource Center (MIDRC), which was expanded to include AI analyses of cancer imaging data.
Developing AI for precision medicine, with a global health perspective
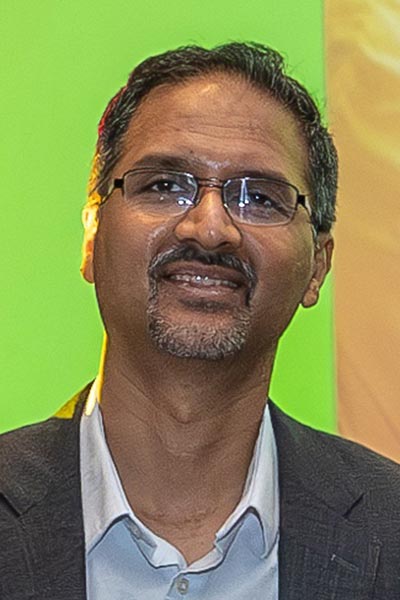
Anant Madabhushi, PhD, professor at the Winship Cancer Institute at Emory University, said, “As we think about AI for precision medicine, we also have to be thinking about it from a global context.”
It is important to think about how these new technologies may benefit patients not just in developed countries but on a worldwide basis, he said, particularly in low- and middle-income countries (LMICs).
Madabhushi described how AI algorithms and data mining applied to whole-slide imaging can help digitally phenotype tissue. He also discussed an AI tool for analyzing computationally derived image features from breast cancer hematoxylin and eosin stain images; this tool improved the predictive capacity of a breast cancer risk-stratification assay. He reviewed recent research evaluating AI-enabled analysis of radiologic features in the tumor milieu to predict treatment response and treatment-related adverse effects.
Madabhushi said that data for diverse populations need to be intentionally incorporated into AI training sets and risk prediction models. He said, “We have to be intentional about equity; it cannot be an afterthought.”
Multiscale AI-facilitated drug discovery at scale, in large patient cohorts
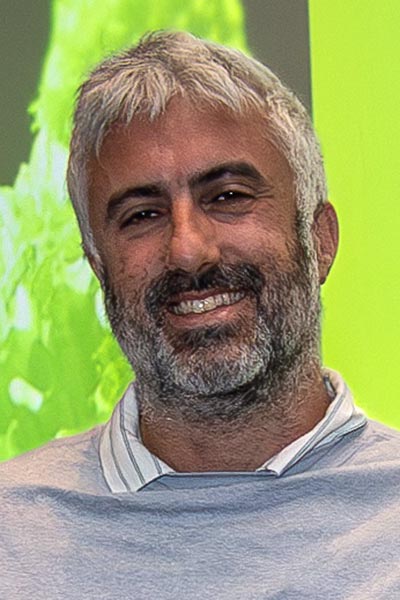
Thomas Clozel, MD, chief executive officer and co-founder at Owkin, framed the problem in precision medicine, stating that precision medicine does not happen at scale today. Only a small proportion of patients with cancer are receiving and benefiting from differentiated personalized precision treatments, he said.
Clozel said that focusing on using expert-curated high-quality patient-level data, rather than high-throughput screens of cell lines, can help uncover new biology and help pair biology in patient subgroups to appropriately matched therapies.
As a sustainable way to access data for large cohorts of patients across hospitals and treatment centers at scale, Clozel said that adopting a federated learning approach—in which data remains local and secure, but the algorithm developed using these data is shared across sites—can help maintain data security and privacy, while also helping improve the performance of AI-enabled clinical tools.
Clozel said, “Causality is what we miss in the field of biology today. We are only talking about correlative biology toward different outcomes.” He said that the battle of using AI to integrate multiscale data and query causality is a worthwhile endeavor.
Leveraging a large dataset to develop a pan-tumor biomarker
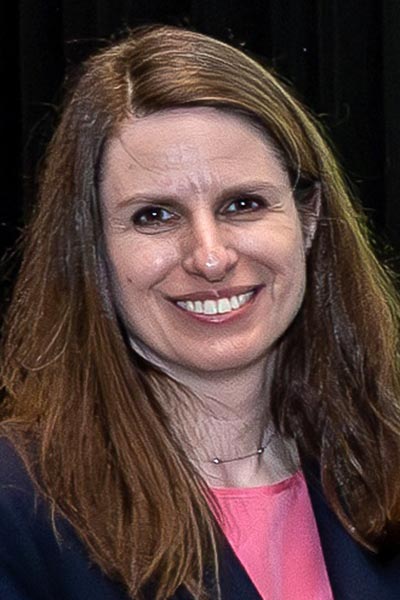
Mia A. Levy, MD, PhD, chief medical officer at Foundation Medicine, Inc., underscored the rigor of validation needed to bring AI technologies from discovery to the bedside for advancing cancer care. She said, “What is important to understand is that even though we use [flashy] terms like AI, anything that we develop using these tools still needs to undergo the rigorous clinical validation, like any other tool that we would use in clinical practice.”
Levy said that access to a curated dataset of comprehensive genomic profiling of tumors derived from a diverse global population, correlated with real-world clinical outcomes, provided a robust platform for biomarker discovery and validation.
Levy reviewed how this dataset was leveraged to identify a new homologous recombination deficiency (HRD) signature. She highlighted how the susceptibility of HRD tumors to poly (ADP-ribose) polymerase (PARP) inhibitor therapy has been leveraged in cancer therapy. HRD affects multiple loci across the cancer genome, resulting in “genomic scars,” she said.
Levy underscored the rationale for developing a pan-tumor HRD signature, as most HRD markers to date were developed for use in ovarian cancer. The pan-tumor HRD signature was developed using a machine-learning genomic-scar based analysis of copy number variation profiles. The signature, HRDSig, was used to identify patients eligible for treatment with a PARP inhibitor.